Project Description
Affiliates
Project Staff
Ideas
Upcoming Events
Curriculum Vitae
Google profile
|
Project Description |
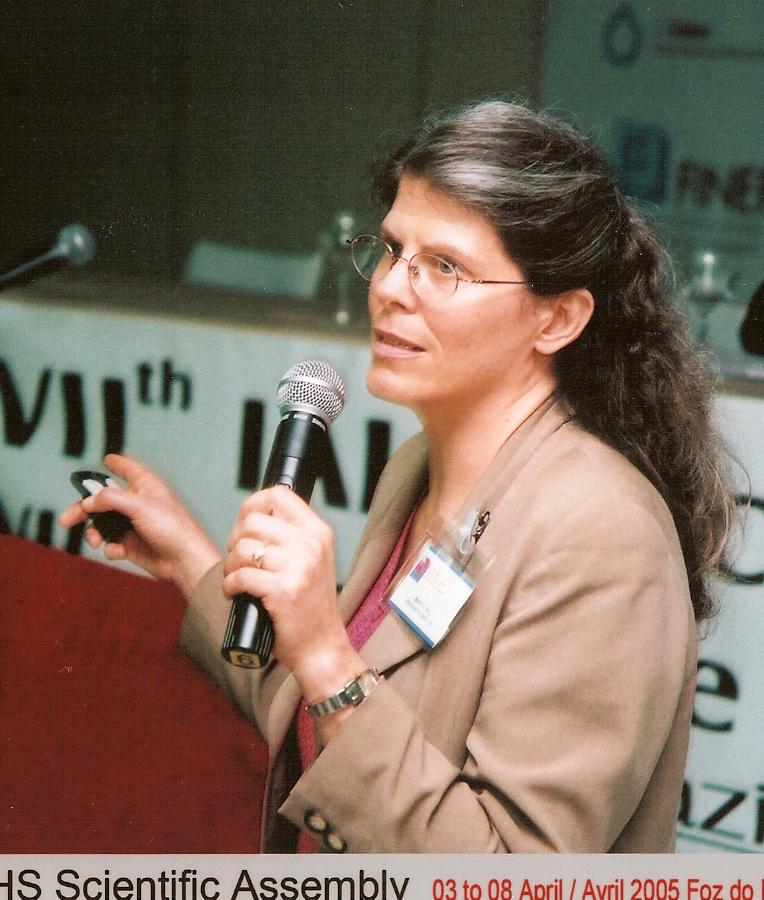
Mary C. Hill, Project Chief, Boulder, Colorado
A fundamental problem in the analysis of complex environmental systems, including groundwater systems, is the interplay of data and modeling, both when testing fundamental theories and when calibrating models. Improving how data and models are used has proven to be exceedingly difficult, however, partly because models are commonly plagued by spurious numerically-based nonlinearities in addition to fundamentally important process-based nonlineairities, and partly because models are not used to as much advantage as they could be. One of the most difficult problems is that though environmental model calibration methods are maturing to include rigorous methods of relating models to the calibration data and the predictions of interest, it is still often unclear how to attain good model predictive ability, as needed for resource planning. A basic question is what level of model detail and parameterization are likely to yield the most accurate predictions. Also, in groundwater models, the use of geologic principles to constrain model characteristics such as the geometry of interconnections and barriers is critical. Yet the geology is often poorly known, leading to groundwater flow patterns that are often grossly in error, and resulting errors in estimated parameter values. This then obscures the ability to derive any general understanding of processes from a set of studies.
This project seeks to improve how data and models are used together to obtain useful system characterization and predictions by improving the transparency and refutability of models. Transparency is improved by creating and evaluating statistics that reveal observations important to parameters, and observations, parameters, and system processes important to predictions and prediction uncertainty. Evaluation of the statistics developed on the project and by others is essential because of the proliferation of such statistics. At this point, nearly every report using a different set of statistics, making it nearly impossible for those using models to compare different modeling efforts. In such an environment transparency is often diminished instead of enhanced by the methods available. Refutability is improved by closely considering data errors and model fit to data to obtain a measure of model error. Evaluation of multiple alternative models is encouraged to test hypotheses and include the views of many stakeholders in the modeling process.
"The methods of science depend on our attempts to describe the world with simple theories. Theories that are complex become unstable, even if they happen to be true.
Science may be described as the art of oversimplification: the art of discerning what we may with advantage omit."
Popper, 1982, The Open Universe: Hutchinson, London, UK. 185p.
Recent News |
Come to the AGU session on model analysis! Conveners include Mary C. Hill (USGS), Ramesh Teegavarapu (FAU), Michael Piasecki (CCNY), Christopher T. Green (USGS), Chandra Pathak (SFWMD), Donald Vasco (LBL), Lindell Ormsby (UKy), Fabio Iwashita (DRI). Session title: Uncertainty Assessment, Optimization, and Sensitivity Analysis in Integrated Hydrologic Modeling as Applications of HydroInformatics |
|
|
|
Recent Publications |
2010-Social and Behavioral Sciences - Identifying important observations using cross validation and computationally frugal sensitivity analysis methods- on-line abstract of journal article |
2010-Journal of Hydrology - Comment on "Two statistics for evaluating parameter identifiability and error reduction" - on-line abstract of journal article |
2010-Ground Water - Ground Water -- Foreword: Groundwater modeling and public policy - Special issues with papers from the MODFLOW 2008 conference |
|
|
|
|
|